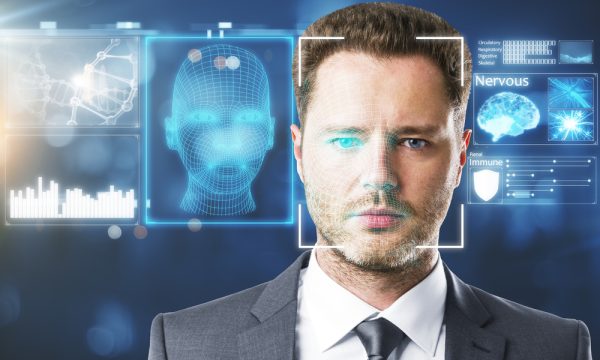
Businessman portrait with digital interface. Face recognition concept. Double exposure
Facial Recognition is an automated process of recognizing a person or persons by their faces. The technology uses biometrics to map facial features from a photograph or video and then compares them to stored facial data for identification purposes. Facial recognition can be used in various forms, including two-factor authentication and real-time tracking systems.
There are three main types of facial recognition – one-to-one verification, one-to-many identification, and active learning algorithms. One-to-one verification involves comparing a user’s input with a single reference image in order to verify the identity of that particular user, whereas one-to-many identification requires matching the inputted image with multiple reference images for accurate identification.
Active learning algorithms use supervised machine learning methods to improve accuracy by continually adapting their parameters based on new data points given as feedback after each iteration of training or testing phases.
How Facial Recognition Works
One of the most common types of facial recognition algorithms is a one-to-one verification algorithm. This type of algorithm compares a user’s input image with a single reference image in order to verify the identity of that particular user.
This type of algorithm is often used for two-factor authentication, allowing users to log into online accounts or services using both their face and password. The accuracy and speed at which this process occurs depend on how accurately the reference images have been captured and stored in the system’s database.
Another common type of facial recognition technology uses one-to-many identification algorithms, which involve matching an inputted image with multiple reference images within its database in order to identify who it belongs to.
These types of systems are typically more accurate than one-to-one verification because they can utilize additional data points from each person’s face such as hair color, and skin tone, or even expressions like smiling or frowning when making comparisons between faces.
However, these systems can be slower since they require comparing against multiple references before arriving at an identification result.
Active learning algorithms use supervised machine learning methods to improve accuracy by continually adapting their parameters based on new data points given as feedback after each iteration of training or testing phases. By doing so, these systems become better equipped over time at recognizing new faces with improved precision and accuracy rates compared to traditional detection methods alone.
Applications of Facial Recognition
Facial recognition technology is being used in a range of applications, including law enforcement, transportation security, and retail.
In the realm of law enforcement, facial recognition can be used to identify suspects from surveillance footage or to cross-check criminal databases for matches. This technology is becoming increasingly popular among police departments as it allows them to quickly compare unknown faces with those already stored in databases such as mugshots and driver’s license photos. It also helps eliminate human error that can occur when manually searching through large amounts of images.
Transportation security has seen an increase in the use of facial recognition technology over recent years due to its ability to provide quick and accurate identification at airports, train stations, and other secure areas. By scanning passengers’ faces against travel documents like passports or visas, this system can help authorities detect fraudsters trying to board planes using false identities or attempting entry into restricted areas without authorization. Additionally, it provides a more efficient alternative than manual checking which often leads to long wait times during peak periods of travel activity.
Retail establishments are also beginning to incorporate facial recognition software into their operations as a way of streamlining customer service processes and improving shoplifting prevention efforts by tracking customers entering the store and monitoring activity throughout the premises via cameras linked up with face detection algorithms. This type of system not only promotes quicker checkout times but can also help staff better understand shopper behavior patterns which could lead to improved marketing strategies down the line too.
Challenges of Facial Recognition
One of the key challenges associated with facial recognition technology is privacy. As this technology becomes more widely used, people increasingly worry about their own data being collected and stored without their knowledge or consent.
Since facial recognition algorithms are trained using large datasets, there is a risk that biases could be built into the system and lead to inaccurate results based on race or gender. Furthermore, with these systems relying heavily on camera footage as input data, they can be vulnerable to spoofing attempts, whereby hackers use photos or videos of someone’s face in order to gain access to secure areas or accounts.
Another challenge of facial recognition involves accuracy issues due to changing environmental conditions such as lighting and angles which can affect the quality of images captured by cameras leading to false matches in some cases. In order for these systems to be effective at accurately identifying individuals from live video streams, they need constant updates and refinements which require significant development resources from both hardware and software companies alike.
Finally, although many countries have laws protecting citizens’ personal information online (such as GDPR), there remain gaps when it comes specifically to how facial recognition technology should be regulated and what rights people have over their biometric identifiers like fingerprints or faces. With governments across the world still trying to arrive at solutions around this issue, it has become an area where progress needs further improvement if we are ever going to reach a consensus on how this powerful tool should responsibly operate within societies today.
Conclusion
Facial recognition technology can provide a number of benefits when used responsibly. It is an effective tool for quickly and accurately verifying identities or detecting fraudsters in various different areas such as law enforcement, transportation security, and retail settings. It can help streamline customer service processes while also providing authorities with more efficient methods to monitor large public spaces.
However, there are potential issues that need to be addressed in order for this technology to be used safely and ethically, such as privacy concerns due to data collection practices and accuracy issues due to changing environmental conditions. The lack of clear regulations on how facial recognition should be managed means that governments across the world still need to take action before this powerful tool can be fully utilized without fear of abuse or misuse.